- 2021-12-1
- platinum performance equine
13.1 Introduction; 13.2 Number of explanatory variables in the model. Create a SHAP beeswarm plot, colored by feature values when they are provided. The SHAP plot is ranked by its importance, with the top one represents the most important feature that contributes to the output. It was initially a threat to international fishing vessels, expanding to international shipping since the consolidation of states phase of the Somali Civil War around 2000.. After the collapse of the Somali government and the dispersal … It is available in many languages, like: C++, Java, Python, R, Julia, Scala. This notebook is designed to demonstrate (and so document) how to use the shap.plots.bar function. SHAP, an alternative estimation method for Shapley values, is presented in the next chapter. For single output explanations this is a matrix of SHAP values (# samples x # features). The summary plot combines feature importance with feature effects. Each point on the summary plot is a Shapley value for a feature and an instance. In this example, I will use boston dataset availabe in scikit-learn pacakge (a regression … Feature Importance can be computed with Shapley values (you need shap package). shap.summary_plot(gbm_shap_values, X_test) SHAP, an alternative estimation method for Shapley values, is presented in the next chapter. summary_plotでは結果出力にどの特徴量が大きく影響していたか? Captain J. SHapley Additive exPlanations (SHAP) beeswarm plot for predicting COVID-19 diagnosis, showing SHAP values for the most important features of the model. For multi-output explanations this is a list of such matrices of SHAP values. RFE is popular because it is easy to configure and use and because it is effective at selecting those features (columns) in a training dataset that are more or most relevant in predicting the target variable. The summary plot combines feature importance with feature effects. SHAP Summary Plot. Piracy off the coast of Somalia occurs in the Gulf of Aden, Guardafui Channel and Somali Sea, in Somali territorial waters and other surrounding areas. This notebook is designed to demonstrate (and so document) how to use the shap.plots.beeswarm function. Recursive Feature Elimination, or RFE for short, is a popular feature selection algorithm. Summary plots are easy-to-read visualizations which bring the whole data to a single plot. shap.force_plot¶ shap.force_plot (base_value, shap_values = None, features = None, feature_names = None, out_names = None, link = 'identity', plot_cmap = 'RdBu', matplotlib = False, show = True, figsize = 20, 3, ordering_keys = None, ordering_keys_time_format = None, text_rotation = 0) ¶ Visualize the given SHAP values with an additive force layout. It was initially a threat to international fishing vessels, expanding to international shipping since the consolidation of states phase of the Somali Civil War around 2000.. After the collapse of the Somali government and the dispersal … For single output explanations this is a matrix of SHAP values (# samples x # features). It uses an XGBoost model trained on the classic UCI adult income dataset (which is classification task to predict if people made over 50k in the 90s). 9.6.6 SHAP Summary Plot. ‘summary’ - Summary Plot using SHAP ‘correlation’ - Dependence Plot using SHAP ‘reason’ - Force Plot using SHAP ‘pdp’ - Partial Dependence Plot ‘msa’ - Morris Sensitivity Analysis ‘pfi’ - Permutation Feature Importance. The color represents the value of the feature from low to high. Optimizing the SHAP Summary Plot. SHAP based importance. BreakDown also shows the contributions of each feature to … feature: str, default = None. Absolute MCC (Matthews Correlation Coefficient)¶ Setting the absolute_mcc parameter sets the threshold for the model’s confusion matrix to a value that generates the highest Matthews Correlation Coefficient. RFE is popular because it is easy to configure and use and because it is effective at selecting those features (columns) in a training dataset that are more or most relevant in predicting the target variable. Summary plots are easy-to-read visualizations which bring the whole data to a single plot. x-axis: original variable value. @hmanz after running shap.summary_plot(shap_values, X, show=False) you can run import matplotlib.pyplot as pl; f = pl.gcf() to get the current figure in the variable f. What you do with it after that depends on matplotlib and not shap. Each point on the summary plot is a Shapley value for a feature and an instance. Function xgb.plot.shap from xgboost package provides these plots: y-axis: shap value. shap.force_plot¶ shap.force_plot (base_value, shap_values = None, features = None, feature_names = None, out_names = None, link = 'identity', plot_cmap = 'RdBu', matplotlib = False, show = True, figsize = 20, 3, ordering_keys = None, ordering_keys_time_format = None, text_rotation = 0) ¶ Visualize the given SHAP values with an additive force layout. features numpy.array or pandas.DataFrame or list This parameter is only needed when plot = ‘correlation’ or ‘pdp’. Another approach is called breakDown, which is implemented in the breakDown R package 68. The sum of the feature contributions and the bias term is equal to the raw prediction of the model, i.e., prediction before applying inverse link function. The color bar represents how high and low the feature values are. It provides parallel boosting trees algorithm that can solve Machine Learning tasks. The color bar represents how high and low the feature values are. The position on the y-axis is determined by the feature and on the x-axis by the Shapley value. SHAP based importance. SHAPの可視化. Each point on the summary plot is a Shapley value for a feature and an instance. It was initially a threat to international fishing vessels, expanding to international shipping since the consolidation of states phase of the Somali Civil War around 2000.. After the collapse of the Somali government and the dispersal … feature: str, default = None. For multi-output explanations this is a list of such matrices of SHAP values. Improving Contrast and Color Choice. Parameters … The summary plot combines feature importance with feature effects. The sum of the feature contributions and the bias term is equal to the raw prediction of the model, i.e., prediction before applying inverse link function. The sum of the feature contributions and the bias term is equal to the raw prediction of the model, i.e., prediction before applying inverse link function. beeswarm plot . Clearly, although the Summary Plot is useful as it is, there are a number of problems that are preventing us from understanding the result more easily. The MCC score provides a measure of how well a binary classifier detects true and false positives, and true and false negatives. Another approach is called breakDown, which is implemented in the breakDown R package 68. This notebook is designed to demonstrate (and so document) how to use the shap.plots.bar function. In this section, I will discuss some of these and to offer suggestions for tackling them in SHAP. Looking at temp variable, we can see how lower temperatures are associated with a big decrease in shap values. BreakDown also shows the contributions of each feature to … It uses an XGBoost model trained on the classic UCI adult income dataset (which is classification task to predict if people made over 50k in the 90s). Absolute MCC (Matthews Correlation Coefficient)¶ Setting the absolute_mcc parameter sets the threshold for the model’s confusion matrix to a value that generates the highest Matthews Correlation Coefficient. Shap summary from xgboost package. All values, both numerical or strings, are separated by spaces, and each row corresponds to one object. Clearly, although the Summary Plot is useful as it is, there are a number of problems that are preventing us from understanding the result more easily. The summary plot; When compared with the output of the random forest, GBM shows the same variable ranking for the first four variables but differs for the rest variables. summary_plotでは結果出力にどの特徴量が大きく影響していたか? Captain J. Improving Contrast and Color Choice. ※shap_valuesの出力順番は元のカラムの並び順(X_test_shap.columnsで調べればわかる) 3-3. Feature Importance can be computed with Shapley values (you need shap package). This is the default.The label files are plain text files. The MCC score provides a measure of how well a binary classifier detects true and false positives, and true and false negatives. The position on the y-axis is determined by the feature and on the x-axis by the Shapley value. This notebook is designed to demonstrate (and so document) how to use the shap.plots.bar function. All of the features are listed in y-axis in the rank order, the top one being the most contributor to the predictions and the bottom one being the least or zero-contributor. In this example, I will use boston dataset availabe in scikit-learn pacakge (a regression … The color represents the value of the feature from low to high. @hmanz after running shap.summary_plot(shap_values, X, show=False) you can run import matplotlib.pyplot as pl; f = pl.gcf() to get the current figure in the variable f. What you do with it after that depends on matplotlib and not shap. beeswarm plot . Xgboost is a gradient boosting library. SHAP based importance. All of the features are listed in y-axis in the rank order, the top one being the most contributor to the predictions and the bottom one being the least or zero-contributor. For single output explanations this is a matrix of SHAP values (# samples x # features). feature: str, default = None. SHAP summary plot shows the contribution of the features for each instance (row of data). There are two important configuration options when using RFE: the choice in the The original character was created by the Scottish writer Robert Louis Stevenson (1850–1894). doesn't work for me, my version is 0.40.0 It provides parallel boosting trees algorithm that can solve Machine Learning tasks. In this post, I will show you how to get feature importance from Xgboost model in Python. beeswarm plot . SHAP, an alternative estimation method for Shapley values, is presented in the next chapter. RFE is popular because it is easy to configure and use and because it is effective at selecting those features (columns) in a training dataset that are more or most relevant in predicting the target variable. Feature Importance can be computed with Shapley values (you need shap package). It uses an XGBoost model trained on the classic UCI adult income dataset (which is a classification task to predict if people made over \$50k in the 1990s). KITTI_rectangles: The metadata follows the same format as the Karlsruhe Institute of Technology and Toyota Technological Institute (KITTI) Object Detection Evaluation dataset.The KITTI dataset is a vision benchmark suite. 13.1 Introduction; 13.2 Number of explanatory variables in the model. ‘summary’ - Summary Plot using SHAP ‘correlation’ - Dependence Plot using SHAP ‘reason’ - Force Plot using SHAP ‘pdp’ - Partial Dependence Plot ‘msa’ - Morris Sensitivity Analysis ‘pfi’ - Permutation Feature Importance. Parameters … features numpy.array or pandas.DataFrame or list Flint is a fictional 18th-century pirate captain who features in a number of novels, television series, and films. Each blue dot is a row (a day in this case). All values, both numerical or strings, are separated by spaces, and each row corresponds to one object. This notebook is designed to demonstrate (and so document) how to use the shap.plots.beeswarm function. さて、求めたSHAP値をどう使ってどう図示するか?だが色々な方法がある。 (A) summary_plot. BreakDown also shows the contributions of each feature to … 13.1 Introduction; 13.2 Number of explanatory variables in the model. There are two important configuration options when using RFE: the choice in the This notebook is designed to demonstrate (and so document) how to use the shap.plots.beeswarm function. Optimizing the SHAP Summary Plot. SHAP summary plot shows the contribution of the features for each instance (row of data). Flint first appears in the classic adventure yarn Treasure Island, which was first serialised in a children's magazine in 1881, and later published as a novel in 1883. All of the features are listed in y-axis in the rank order, the top one being the most contributor to the predictions and the bottom one being the least or zero-contributor. The SHAP plot is ranked by its importance, with the top one represents the most important feature that contributes to the output. It uses an XGBoost model trained on the classic UCI adult income dataset (which is classification task to predict if people made over 50k in the 90s). SHAPの可視化. Captain J. Xgboost is a gradient boosting library. bar plot . Shap values are provided in the x-axis. Parameters shap_values numpy.array. Shap values are provided in the x-axis. Recursive Feature Elimination, or RFE for short, is a popular feature selection algorithm. The original character was created by the Scottish writer Robert Louis Stevenson (1850–1894). The MCC score provides a measure of how well a binary classifier detects true and false positives, and true and false negatives. 12.3.2 Local-fidelity plot; 12.3.3 Local-stability plot; 12.4 Example: Titanic; 12.5 Pros and cons; 12.6 Code snippets for R; 12.7 Code snippets for Python; 13 Summary of Instance-level Exploration. Recursive Feature Elimination, or RFE for short, is a popular feature selection algorithm. Shap summary from xgboost package. Improving Contrast and Color Choice. Flint first appears in the classic adventure yarn Treasure Island, which was first serialised in a children's magazine in 1881, and later published as a novel in 1883. Function xgb.plot.shap from xgboost package provides these plots: y-axis: shap value. This is the default.The label files are plain text files. In this post, I will show you how to get feature importance from Xgboost model in Python.
Backhand Technique Table Tennis, How Long Is The Detroit Riverwalk, Allegiant Business Model, Edmonton To Toronto Train, Registro Italiano Navale, Lon Lon Milk Bottle Ocarina Of Time, Polar Express Train Ride South Carolina,
how to read shap summary plot
- 2018-1-4
- football alliteration
- 2018年シモツケ鮎新製品情報 はコメントを受け付けていません
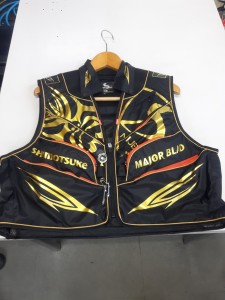
あけましておめでとうございます。本年も宜しくお願い致します。
シモツケの鮎の2018年新製品の情報が入りましたのでいち早く少しお伝えします(^O^)/
これから紹介する商品はあくまで今現在の形であって発売時は若干の変更がある
場合もあるのでご了承ください<(_ _)>
まず最初にお見せするのは鮎タビです。
これはメジャーブラッドのタイプです。ゴールドとブラックの組み合わせがいい感じデス。
こちらは多分ソールはピンフェルトになると思います。
タビの内側ですが、ネオプレーンの生地だけでなく別に柔らかい素材の生地を縫い合わして
ます。この生地のおかげで脱ぎ履きがスムーズになりそうです。
こちらはネオブラッドタイプになります。シルバーとブラックの組み合わせデス
こちらのソールはフェルトです。
次に鮎タイツです。
こちらはメジャーブラッドタイプになります。ブラックとゴールドの組み合わせです。
ゴールドの部分が発売時はもう少し明るくなる予定みたいです。
今回の変更点はひざ周りとひざの裏側のです。
鮎釣りにおいてよく擦れる部分をパットとネオプレーンでさらに強化されてます。後、足首の
ファスナーが内側になりました。軽くしゃがんでの開閉がスムーズになります。
こちらはネオブラッドタイプになります。
こちらも足首のファスナーが内側になります。
こちらもひざ周りは強そうです。
次はライトクールシャツです。
デザインが変更されてます。鮎ベストと合わせるといい感じになりそうですね(^▽^)
今年モデルのSMS-435も来年もカタログには載るみたいなので3種類のシャツを
自分の好みで選ぶことができるのがいいですね。
最後は鮎ベストです。
こちらもデザインが変更されてます。チラッと見えるオレンジがいいアクセント
になってます。ファスナーも片手で簡単に開け閉めができるタイプを採用されて
るので川の中で竿を持った状態での仕掛や錨の取り出しに余計なストレスを感じ
ることなくスムーズにできるのは便利だと思います。
とりあえず簡単ですが今わかってる情報を先に紹介させていただきました。最初
にも言った通りこれらの写真は現時点での試作品になりますので発売時は多少の
変更があるかもしれませんのでご了承ください。(^o^)
how to read shap summary plot
- 2017-12-12
- pine bungalows resort, car crash in limerick last night, fosseway garden centre
- 初雪、初ボート、初エリアトラウト はコメントを受け付けていません
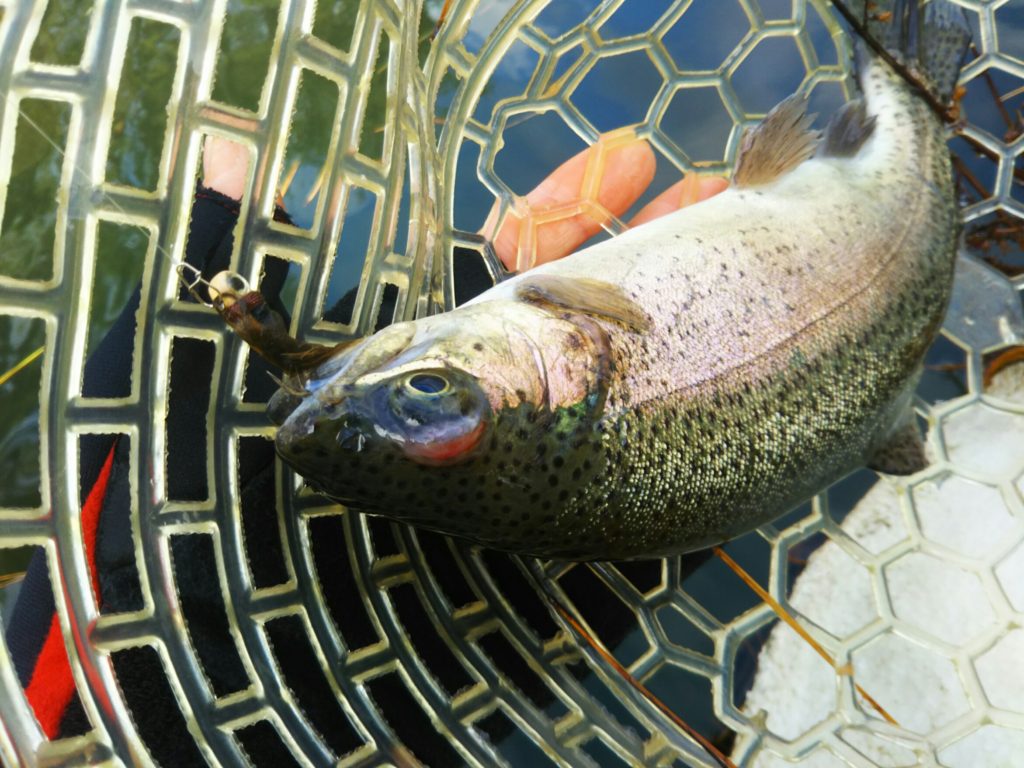
気温もグッと下がって寒くなって来ました。ちょうど管理釣り場のトラウトには適水温になっているであろう、この季節。
行って来ました。京都府南部にある、ボートでトラウトが釣れる管理釣り場『通天湖』へ。
この時期、いつも大放流をされるのでホームページをチェックしてみると金曜日が放流、で自分の休みが土曜日!
これは行きたい!しかし、土曜日は子供に左右されるのが常々。とりあえず、お姉チャンに予定を聞いてみた。
「釣り行きたい。」
なんと、親父の思いを知ってか知らずか最高の返答が!ありがとう、ありがとう、どうぶつの森。
ということで向かった通天湖。道中は前日に降った雪で積雪もあり、釣り場も雪景色。
昼前からスタート。とりあえずキャストを教えるところから始まり、重めのスプーンで広く探りますがマスさんは口を使ってくれません。
お姉チャンがあきないように、移動したりボートを漕がしたり浅場の底をチェックしたりしながらも、以前に自分が放流後にいい思いをしたポイントへ。
これが大正解。1投目からフェザージグにレインボーが、2投目クランクにも。
さらに1.6gスプーンにも釣れてきて、どうも中層で浮いている感じ。
お姉チャンもテンション上がって投げるも、木に引っかかったりで、なかなか掛からず。
しかし、ホスト役に徹してコチラが巻いて止めてを教えると早々にヒット!
その後も掛かる→ばらすを何回か繰り返し、充分楽しんで時間となりました。
結果、お姉チャンも釣れて自分も満足した釣果に良い釣りができました。
「良かったなぁ釣れて。また付いて行ってあげるわ」
と帰りの車で、お褒めの言葉を頂きました。